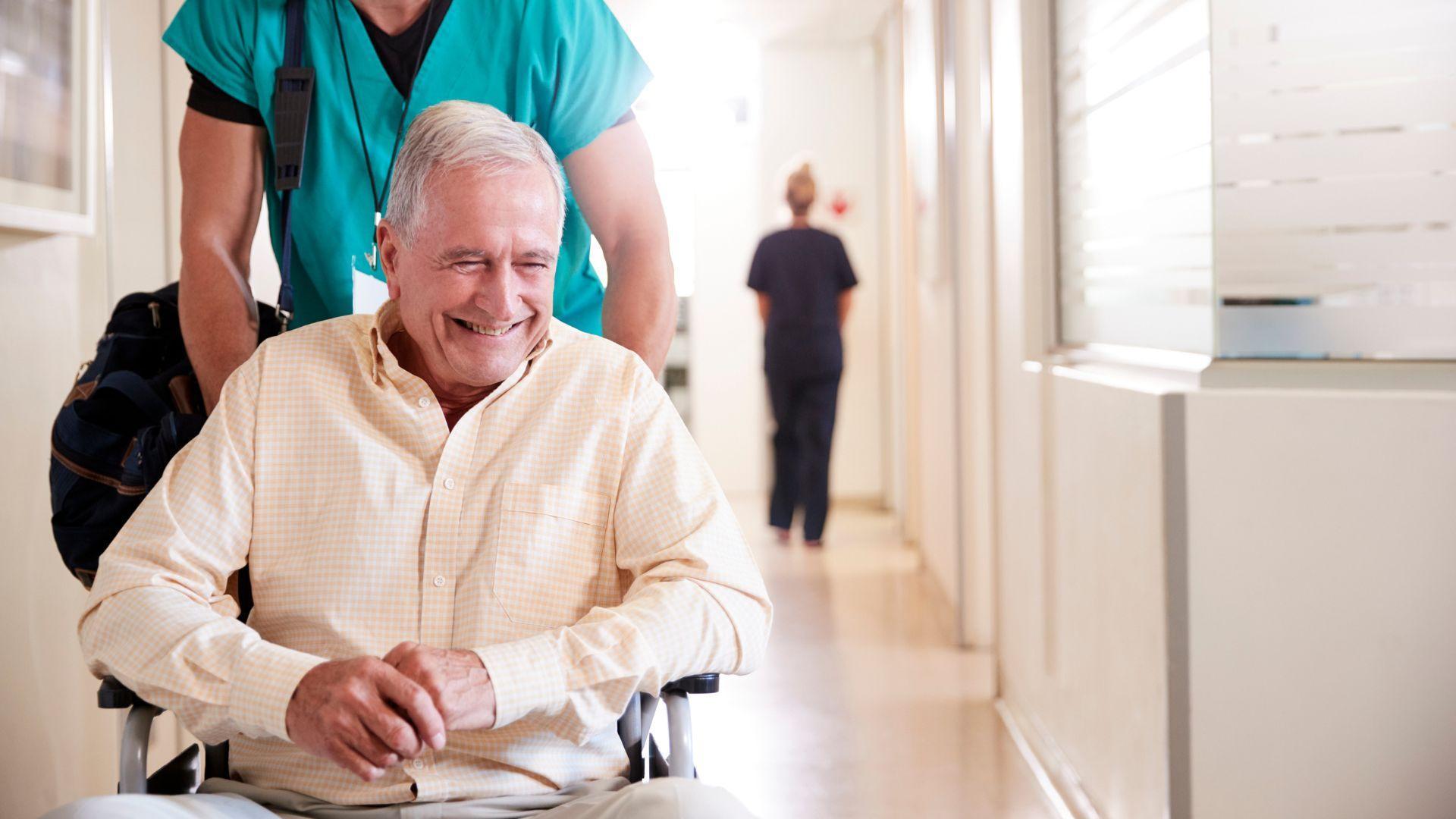
Bridges-2-powered AI matches life-critical performance of other AI and non-AI alternatives — unlike them, its “thinking” is understandable to humans
Artificial intelligence (AI) has transformed our lives, giving us a tool for vastly better-informed decision making. But a decision based on the hidden computations within an AI won’t quite do when human lives and health depend on it. A team of scientists from the nonprofit Mederrara Research, the startup company Sound Prediction Inc., and the National Institutes of Health (NIH) used the Bridges-2 system at PSC to create methodologies for a “transparent AI” whose “thinking process” can truly be understood by humans.
WHY IT’S IMPORTANT
We seldom stop to think about it, but whether we’re searching for what vacuum cleaner to buy, reading incoming social media or feeling our car stop sharply as the automatic braking kicks in, it’s all about AI stepping in and helping us make a complicated decision simpler. AI has done some amazing things for us.
You’re waiting for the downside, though, and here it is: Often we have no idea how or why it’s doing what it’s doing. That makes it extremely difficult to reduce risks or correct biases stemming from AI. A product of deep learning, in which a computer model learns from millions of repetitions of trial and error, modern AI comes up with a great solution by brute force. Much of the time we don’t know why it’s making the decisions it’s making. But when the AI is helping us to decide whether a patient being discharged from a hospital will have a life-threatening relapse, the consequences are deadly serious, and we need to understand how it made its predictions in order to trust that it is working reliably. That way, it can help us pinpoint effective interventions
“It’s hugely challenging, and I think the issue is that … you have a large number of interrelated variables that you think might bias the outcome … [With] these newer models, you can’t accurately track how the prediction is computed from the predictors”. —Josh Chang, Mederrata Research
Mederrata Research is a nonprofit working on reducing medical error. Sound Prediction Inc. is a company focused on making AI’s decisions understandable. Together, they comprised one of 25 teams chosen by the Centers for Medicare & Medicaid Services for their inaugural AI challenge. As part of that competition, Josh Chang of Mederrata and Sound Prediction worked with colleagues there and at the National Institutes of Health to design a way of mimicking properties of AI methods within multilevel Bayesian models – a sophisticated kind of statistical model – that are easy to understand by humans. To develop this software, they turned to the advanced research computer Bridges-2 at PSC.
HOW PSC HELPED
The problem with conventional AI such as deep learning is that the connection between the predictors, the factors that the AI is using to predict outcomes, their interactions with one another and their connections with the prediction are buried deep in the program. Scientists have used so-called “post-hoc explainer” software to try to detangle the AI’s “thinking.” But this is 20/20 hindsight. It gives a “just so” answer that seems to explain the result but usually can’t help us understand the next answer.
Instead, the team would develop a mulitlevel model (MLM) — an advanced statistical tool that shares similarities with many AI programs. Inspired by properties of deep learning, they would use data to separate out cases into cohorts of similar cases. They would then use the MLM to have the machine identify for each cohort a limited number of factors. Once it came up with its answers, a human expert could look at those factors and understand how it was making decisions.
Unlike a classic supercomputing problem — like airflow over an aircraft frame — their AI would need to carry out many parallel but linked decision-based computations rather than solve complicated differential equations. This requirement is a strength of graphics processing units (GPUs). The National Science Foundation-funded Bridges-2 advanced research computer offered powerful next-generation GPUs in abundance. The scientists also needed to do this in a way that held huge chunks of data close to the computation. That was a strength of Bridges-2’s extreme memory nodes. Finally, making this pivot inside the machine between different nodes work — I/O, the rate of moving data “in and out” — was a further strength of Bridges-2.
“What we really like about Bridges is [that] the I/O speeds are very fast. The scheduling [of the computations] also works very well. Things ran much faster at PSC than on equivalent [commercial] systems.” —Josh Chang, Mederrata
The team designed their AI to reveal risk factors for unplanned readmission or death among Medicare patients any time after discharge from the hospital. They started by training the AI on hospital visits for Medicare patients between 2009 and 2011. Then they tested it on data from 2012.
The team ran their transparent AI against the best competition they could find. They ran deep neural networks and ensemble gradient boosted trees — two popular examples of completely sealed-off, non-transparent AI. Finally, they used a post-hoc explainer on their transparent model to compare its given explanation against the model’s true explanation.
The MLM AI’s performance compared well to the other methods. Its AUROC — a measure of the tradeoff between correctly identifying positive predictors of readmission and not accidentally identifying incorrect predictors, with 1 being a perfect score — for 30-day readmission or death was 0.76. Its 90-day AUROC was a little better, 0.78. These were about the same as the AUROCs for the other methods.
Importantly, the post-hoc explainer from the other methods gave convincing yet incorrect explanations for how the AI was deciding. By comparison, the MLM AI’s results were both interpretable and understandable, relating directly to relationships that made sense medically. For example, the AI identified that patients that were discharged to their homes (as opposed to other care settings) were much less likely to be readmitted or die. This observation is an example of Simpson’s paradox. This bias happens when the likelihood of an intervention is correlated with an outcome. In other words, these patients were not having better outcomes because they were discharged to their homes but because they were less sick to begin with. The MLM AI correctly identified this.
Due to the transparent nature of their MLM AI, the Mederrata team was able both to see this bias and adjust for it – not just for a single patient, but also for groups of patients as outlined in the model. By allowing the prediction model to vary by cohort, and forcing the definition of cohorts to use only a few factors, the model is easier to apply to decision making in practice than if a doctor only has case-by-case explanations. The model is also more specific than classical regression methods – simple statistical prediction of outcomes without AI. This allows it to give better guidance in matching patients to effective treatments given limited resources.
The team reported their results at the AI for Social Good workshop at the Association for the Advancement of Artificial Intelligence conference in Washington D.C. in February 2023. They’re aiming for performant models that forecast health and utilization more broadly while still returning results that humans can make sense of.