Bridges Refines Protein Simulations, Approaching Lab Accuracy
To understand how the tiny machinery of life works in health and disease, scientists need accurate pictures of how proteins fold and move. But laboratory methods for imaging proteins are slow, and so the structures of hundreds of thousands of proteins that have been discovered are still unknown. Scientists have used a number of methods for predicting structures via computer simulation. But sometimes even high-quality simulations aren’t as accurate as drug designers may want. A Michigan State University team used the GPU nodes in PSC’s Bridges supercomputer to optimize predictions made by other scientists. In the process, they made predictions of the structures for a number of proteins with accuracy that approached the most precise, X-ray based lab measurements.
Why It’s Important
One of the most important advances in how scientists think about how the contents of our bodies’ cells work is that they no longer imagine proteins as stationary ball-and-stick models. Instead, they see the molecules as ever-moving structures. Understanding how proteins move has opened a window on exactly how they work—and how various disease processes interfere with those movements.
The problem is that there are so many proteins. Humans alone have more than 30,000 different types of protein in their cells. And to understand human health, we also need to understand tens or hundreds of thousands more, in viruses, bacteria and parasites. Scientists have a number of tools—the most precise being X-ray crystallography—for taking snapshots of a given protein’s structure. But these methods are relatively slow, and simply can’t catch up with the backlog of unknown protein structures.
“Proteins carry out biological functions. You can measure and analyze those functions, but to really understand them you have to look at the details of how proteins operate … We know a lot of structures from experiments, but haven’t [done this, for example, with] most of the proteins in bacteria. We need to fill the gap by generating models quickly and efficiently.”—Michael Feig, Michigan State University
To solve the problem of too few known protein structures, scientists have used computer modeling of what a protein might look like. But these methods aren’t always as precise as some scientists need for tasks like designing new medicines.
To address this issue, Lim Heo, a postdoctoral fellow at Michigan State University (MSU) and his advisor Michael Feig turned to molecular dynamics (MD) refinements using the graphics processing unit (GPU) nodes of PSC’s Bridges supercomputer.
How PSC Helped
One problem with even some quite good protein-structure simulations, the Michigan State scientists realized, is that the proteins in them haven’t quite settled into their lowest-energy forms. A person easing into a recliner may need to wiggle a bit to find the most comfortable position. In a similar way, a protein that isn’t folded quite right needs to nudge itself to find a position that doesn’t require unnecessary physical energy. Scientists understand the physics that makes the links between atoms in a protein twist and vibrate. MD simulation uses these rules to refine the thousands of such links in a given protein. Over a long enough simulated time period, the whole structure settles into its least-energy position. But such a simulation takes massive computing power.
GPUs, or graphics processing units—which were originally developed to create better pictures in video games—are ideal for MD simulations. But the team simply couldn’t get hold of enough GPU nodes on the computers initially available to them. Bridges—particularly its advanced NVIDIA P100 GPUs—proved ideal for giving them the power they needed to optimize the previous predictions.
“We’ve demonstrated that in some cases we can reach experimental-structure [accuracy], but can’t quite do it often enough or fast enough. Ideally, we would push a button and get a structure in a day or so. Today, it takes a week to get an answer … We’re working on better methods for crossing barriers and finding the lowest energy state. If we know what’s hindering improvement, we can target that.”—Michael Feig, Michigan State University
The molecular modeling community offered the scientists an opportunity to test their predictions. At the 13th Critical Assessment of Techniques for Protein Structure Prediction (CASP13) competition in 2018, other scientists used a simulation technique called machine learning (ML) to predict the structures of 27 whole or partial proteins that had recently been determined by lab methods.
The MSU team then took these predictions the other scientists had made and refined them using their MD method. Using the same scoring method as in the CASP13 competition, they found that MD improved the ML predictions across the board—their refinements improved the earlier predictions by 3 to 30 percent. In some cases, the MD predictions achieved a precision that rivaled that of the best laboratory imaging methods. Next, the team will work on improving the efficiency of their calculations so that they can simulate longer time periods. Longer simulations allow better refinement, because proteins in an almost-correct initial model may need additional time to settle into their proper shapes. The team reported their latest results in the journal Proteins: Structure, Function and Bioinformatics in November 2019.
You can read the paper here.
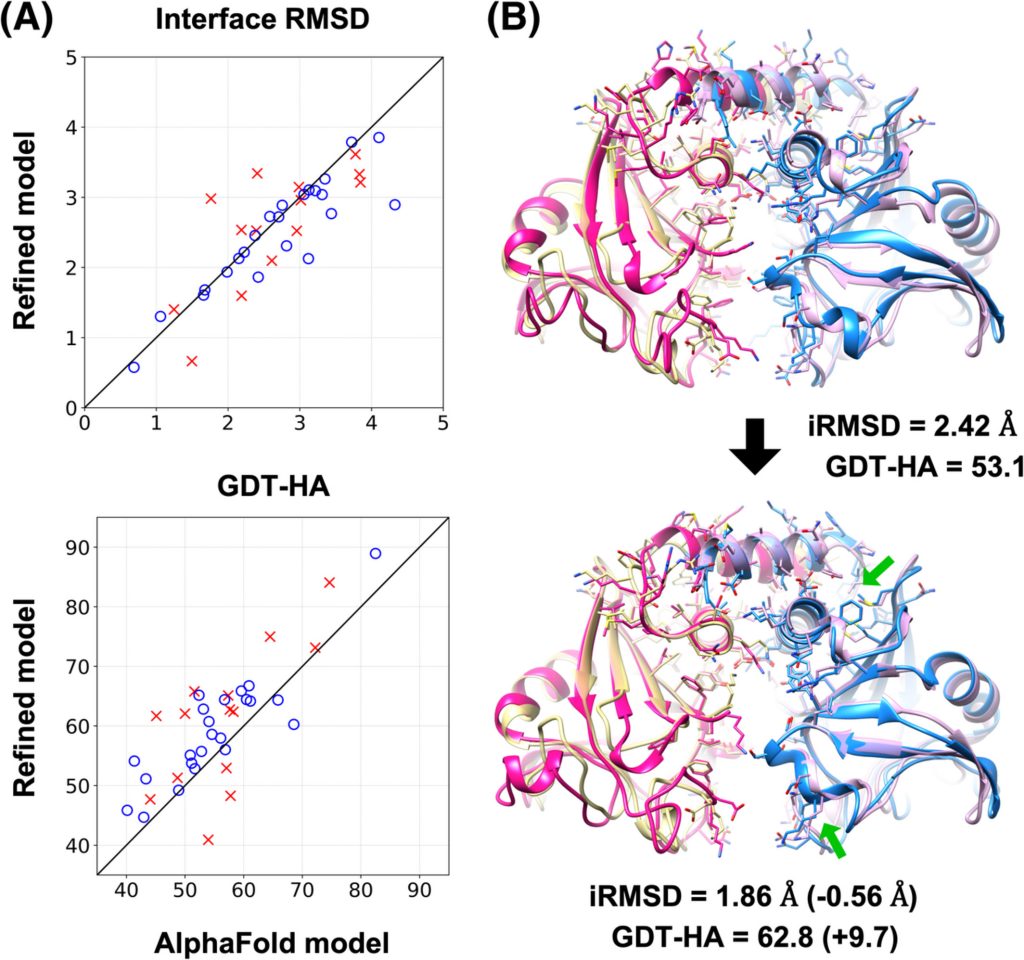