Research at PSC
Advancing science for a better future
PSC staff performs research in many areas of high performance computing, including biomedical applications, networking, scientific applications and cybersecurity.
Biomedical Applications
The Biomedical Applications Group pursues cutting-edge research in high performance computing in the biomedical sciences. They foster collaboration between PSC experts in computational science and biomedical researchers nationwide.
Members of this group develop computational methods and tools and conduct research on biomedical systems at the cell and tissue level with a focus on neural systems, such as the brain and the central nervous system.
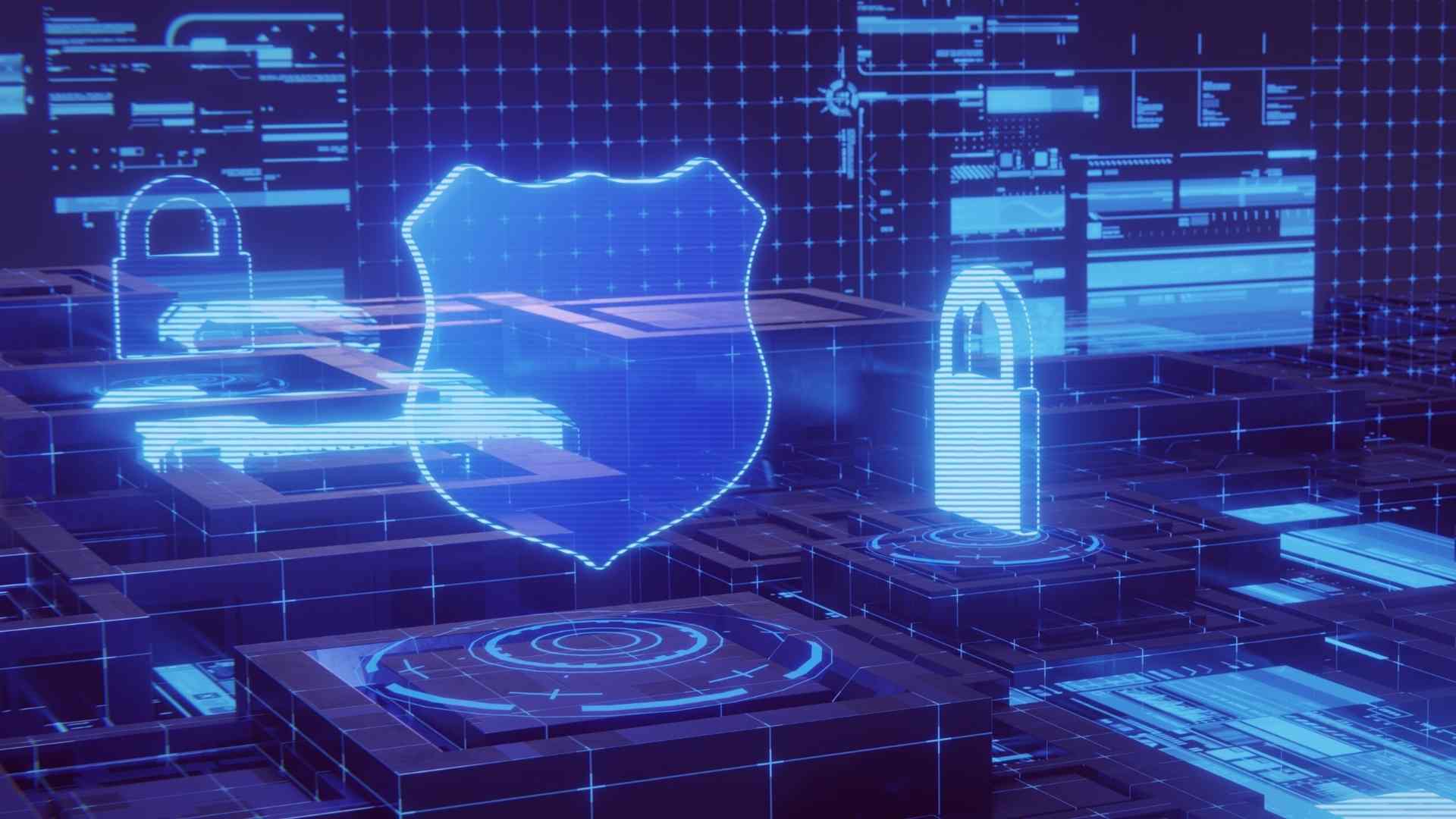
Cybersecurity
PSC is a collaborating institution in the NSF-funded Cybersecurity Group. Part of the Research Security Operations Center, we help provide the research and education community with the cybersecurity services, training and information sharing necessary to make scientific computing resilient to cyberattacks.
High Performance Networking
The Advanced Networking Group conducts research on network performance and analysis in support of high performance computing applications. They also develop software to support heterogeneous distributed supercomputing applications and to implement high-speed interfaces to archival and mass storage systems.
HPC AI and Big Data Group
The Artificial Intelligence and Big Data group converges Artificial Intelligence and high performance computing capabilities, empowering research to grow beyond prevailing constraints.
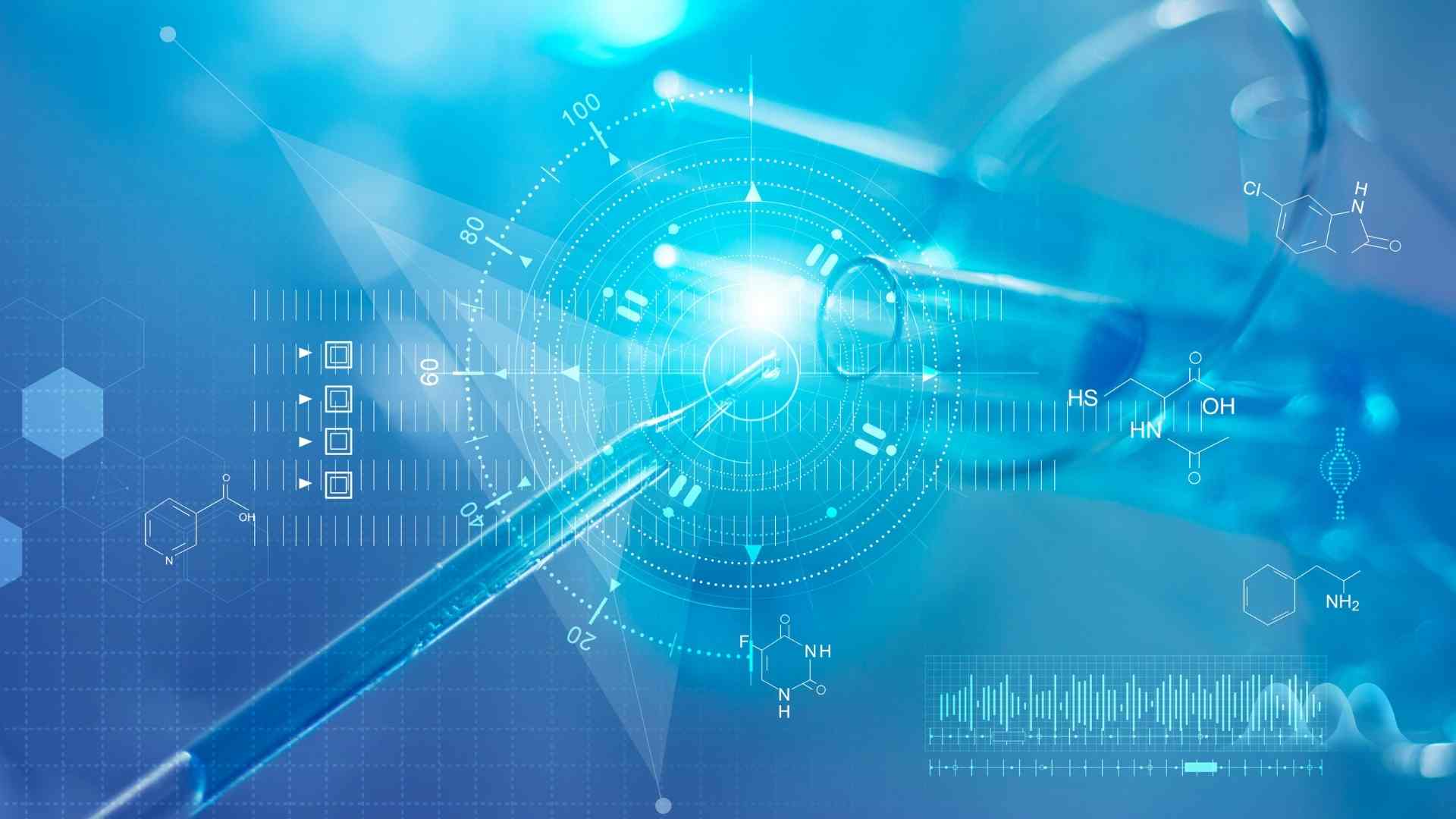
Scientific Applications
The Support for Scientific Applications Group at PSC promotes groundbreaking scientific research through efficient and inventive use of PSC resources. This group often collaborates on research projects to provide expertise in scientific computing.